Remaining Useful Life (RUL) is a critical concept in the world of predictive maintenance, asset management, and reliability engineering. Whether you are managing industrial equipment, vehicles, or even consumer electronics, understanding RUL can significantly enhance operational efficiency and reduce costs. In this comprehensive guide, we will explore the intricacies of RUL, its importance, methodologies, and real-world applications. By the end of this article, you will have a thorough understanding of how RUL works and how it can be applied to various industries.
As industries increasingly adopt predictive maintenance strategies, the role of RUL has become more prominent. It helps businesses anticipate failures, optimize maintenance schedules, and extend the lifespan of their assets. This guide will not only explain the theoretical aspects of RUL but also provide actionable insights and practical examples to help you implement it effectively. Whether you're a professional in the field or simply curious about this topic, this article will serve as your go-to resource for all things RUL.
Before diving into the specifics, it's essential to understand why RUL is a game-changer for modern industries. With the advent of the Internet of Things (IoT), machine learning, and big data analytics, RUL has become more accurate and accessible than ever before. These technologies allow for real-time monitoring and analysis, enabling businesses to make informed decisions about their assets. In the following sections, we will explore the key components of RUL, its methodologies, and how it can be applied in various contexts.
Read also:Exploring The World Of Mad Island Mods Your Ultimate Guide
Table of Contents
- What is RUL?
- The Importance of RUL in Modern Industries
- Key Methodologies for Calculating RUL
- Real-World Applications of RUL
- Challenges in Implementing RUL
- Tools and Technologies for RUL Analysis
- Case Studies: Success Stories of RUL Implementation
- Future Trends in RUL
- Best Practices for Maximizing RUL Benefits
- Conclusion
What is RUL?
Remaining Useful Life (RUL) refers to the estimated time left before an asset or component is expected to fail or require replacement. It is a predictive metric used in maintenance and reliability engineering to determine when an asset should be serviced or retired. By calculating RUL, businesses can avoid unexpected breakdowns, reduce downtime, and optimize maintenance schedules.
RUL is typically expressed in units of time, such as hours, days, or months, depending on the context. For example, in the aviation industry, RUL might be measured in flight hours, while in manufacturing, it could be measured in operational cycles. The goal of RUL is to provide a proactive approach to asset management, enabling organizations to take preventive actions before failures occur.
Key Components of RUL
To fully understand RUL, it's important to break down its key components:
- Data Collection: Gathering data from sensors, historical records, and other sources to monitor the condition of an asset.
- Data Analysis: Using statistical models, machine learning algorithms, and other analytical tools to process the collected data.
- Prediction Models: Applying predictive models to estimate the remaining useful life of an asset based on the analyzed data.
- Decision-Making: Using the RUL predictions to make informed decisions about maintenance, replacement, or retirement of assets.
The Importance of RUL in Modern Industries
In today's fast-paced industrial landscape, downtime can be costly. Whether it's a manufacturing plant, a fleet of vehicles, or a power generation facility, unexpected failures can lead to significant financial losses, safety risks, and reputational damage. This is where RUL comes into play, offering a proactive solution to these challenges.
By accurately predicting the remaining useful life of assets, businesses can:
- Reduce unplanned downtime and maintenance costs.
- Optimize the use of resources and extend the lifespan of assets.
- Enhance safety by preventing catastrophic failures.
- Improve operational efficiency and productivity.
For example, in the aviation industry, RUL is used to monitor the health of aircraft engines. By predicting when an engine is likely to fail, airlines can schedule maintenance during non-peak hours, minimizing disruptions and ensuring passenger safety.
Read also:Unveiling The Wealth Empire Mo Gawdats Net Worth And Inspiring Journey
Why RUL Matters for YMYL Industries
RUL is particularly critical for industries that fall under the YMYL (Your Money or Your Life) category. These industries, such as healthcare, finance, and transportation, have a direct impact on people's lives and well-being. For instance, in healthcare, RUL can be used to monitor medical equipment like MRI machines or ventilators. Ensuring these devices remain operational can be a matter of life and death.
Similarly, in the financial sector, RUL can be applied to IT infrastructure to prevent system failures that could lead to data breaches or financial losses. By prioritizing RUL, YMYL industries can uphold their commitment to safety, reliability, and trustworthiness.
Key Methodologies for Calculating RUL
Calculating RUL involves a combination of data-driven techniques and predictive models. Below are some of the most commonly used methodologies:
1. Physics-Based Models
Physics-based models rely on the underlying physical principles governing the behavior of an asset. These models use equations and simulations to predict how an asset will degrade over time. For example, in the automotive industry, physics-based models can simulate the wear and tear of engine components to estimate their RUL.
2. Data-Driven Models
Data-driven models leverage historical and real-time data to predict RUL. These models use machine learning algorithms, such as regression, neural networks, and decision trees, to analyze patterns and trends in the data. For instance, in manufacturing, data-driven models can analyze sensor data from production lines to predict when a machine is likely to fail.
3. Hybrid Models
Hybrid models combine the strengths of physics-based and data-driven approaches. By integrating physical principles with data analytics, these models provide more accurate and robust RUL predictions. For example, in the energy sector, hybrid models can be used to predict the RUL of wind turbines by combining physical simulations with real-time sensor data.
Real-World Applications of RUL
RUL has a wide range of applications across various industries. Below are some examples of how RUL is being used in real-world scenarios:
1. Aviation
In the aviation industry, RUL is used to monitor the health of critical components such as engines, landing gear, and avionics systems. By predicting when these components are likely to fail, airlines can schedule maintenance proactively, reducing the risk of in-flight failures and ensuring passenger safety.
2. Manufacturing
In manufacturing, RUL is applied to production equipment such as conveyor belts, robotic arms, and CNC machines. By predicting the RUL of these assets, manufacturers can optimize their maintenance schedules, reduce downtime, and improve overall productivity.
3. Healthcare
In healthcare, RUL is used to monitor medical devices such as imaging equipment, surgical tools, and patient monitoring systems. Ensuring these devices remain operational is crucial for delivering high-quality care and avoiding life-threatening situations.
Challenges in Implementing RUL
While RUL offers numerous benefits, implementing it effectively can be challenging. Below are some of the common challenges businesses face when adopting RUL:
- Data Quality: Accurate RUL predictions depend on high-quality data. Incomplete or inaccurate data can lead to unreliable predictions.
- Complexity: Developing and deploying RUL models can be complex, requiring expertise in data science, engineering, and domain knowledge.
- Cost: Implementing RUL solutions can be expensive, especially for small and medium-sized businesses.
- Integration: Integrating RUL models with existing systems and workflows can be challenging, requiring significant changes to processes and infrastructure.
Tools and Technologies for RUL Analysis
Several tools and technologies are available to support RUL analysis. Below are some of the most popular ones:
1. IoT Platforms
IoT platforms enable real-time data collection from sensors and devices, providing the foundation for RUL analysis. Examples include Microsoft Azure IoT, AWS IoT, and IBM Watson IoT.
2. Machine Learning Frameworks
Machine learning frameworks such as TensorFlow, PyTorch, and Scikit-learn are widely used for developing predictive models for RUL.
3. Predictive Maintenance Software
Predictive maintenance software solutions, such as GE Digital's Predix and SAP Predictive Maintenance, offer built-in RUL capabilities, making it easier for businesses to implement and manage RUL models.
Case Studies: Success Stories of RUL Implementation
To illustrate the practical benefits of RUL, let's look at some real-world success stories:
Case Study 1: Predictive Maintenance in Aviation
A major airline implemented RUL models to monitor the health of its aircraft engines. By analyzing sensor data and using machine learning algorithms, the airline was able to predict engine failures with high accuracy. This proactive approach reduced unplanned maintenance by 30% and saved millions of dollars in operational costs.
Case Study 2: RUL in Manufacturing
A leading manufacturing company used RUL to optimize the maintenance of its production equipment. By predicting when machines were likely to fail, the company reduced downtime by 25% and increased overall productivity by 15%.
Future Trends in RUL
The field of RUL is constantly evolving, driven by advancements in technology and increasing demand for predictive maintenance solutions. Below are some of the key trends shaping the future of RUL:
- AI and Machine Learning: AI and machine learning will continue to play a crucial role in improving the accuracy and reliability of RUL predictions.
- Edge Computing: Edge computing will enable real-time RUL analysis at the source, reducing latency and improving response times.
- Digital Twins: Digital twin technology will allow businesses to create virtual replicas of their assets, enabling more accurate RUL predictions.
Best Practices for Maximizing RUL Benefits
To get the most out of RUL, businesses should follow these best practices:
- Invest in high-quality data collection and management systems.
- Collaborate with experts in data science and engineering to develop robust RUL models.
- Regularly update and refine RUL models to ensure accuracy and relevance.
- Integrate RUL solutions with existing workflows and systems to maximize efficiency.
Conclusion
Remaining Useful Life (RUL) is a powerful tool for predictive maintenance and asset management. By accurately predicting when an asset is likely to fail, businesses can reduce downtime, optimize resources, and improve operational efficiency. Whether you're in aviation, manufacturing, healthcare, or any other industry, RUL can help you stay ahead of potential failures and make informed decisions.
We hope this guide has provided you with a comprehensive understanding of RUL and its applications. If you found this article helpful, please share it with your colleagues and leave a comment below. For more insights into predictive maintenance and asset management, explore our other articles on the topic.
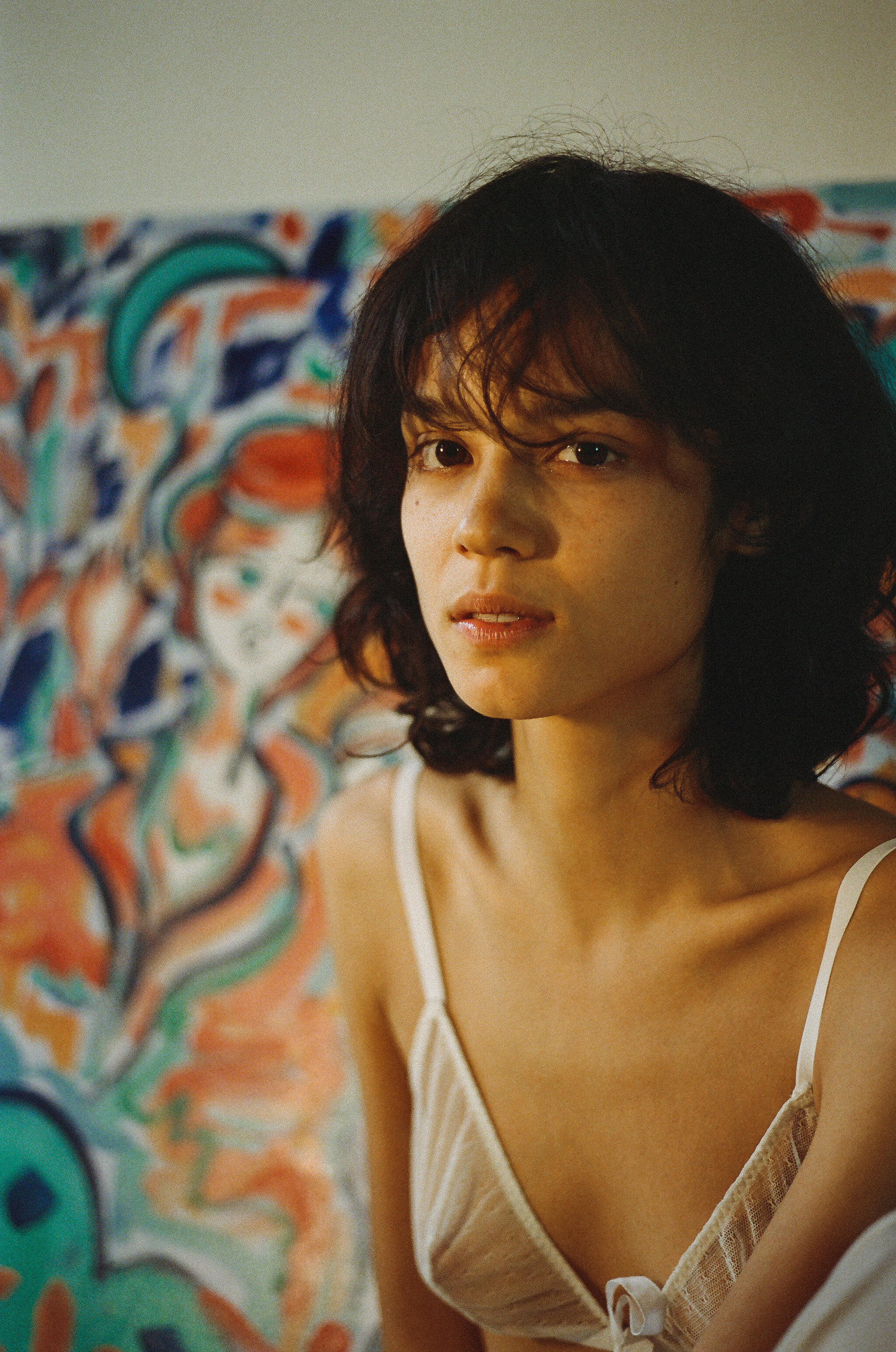.jpg?format=1500w)
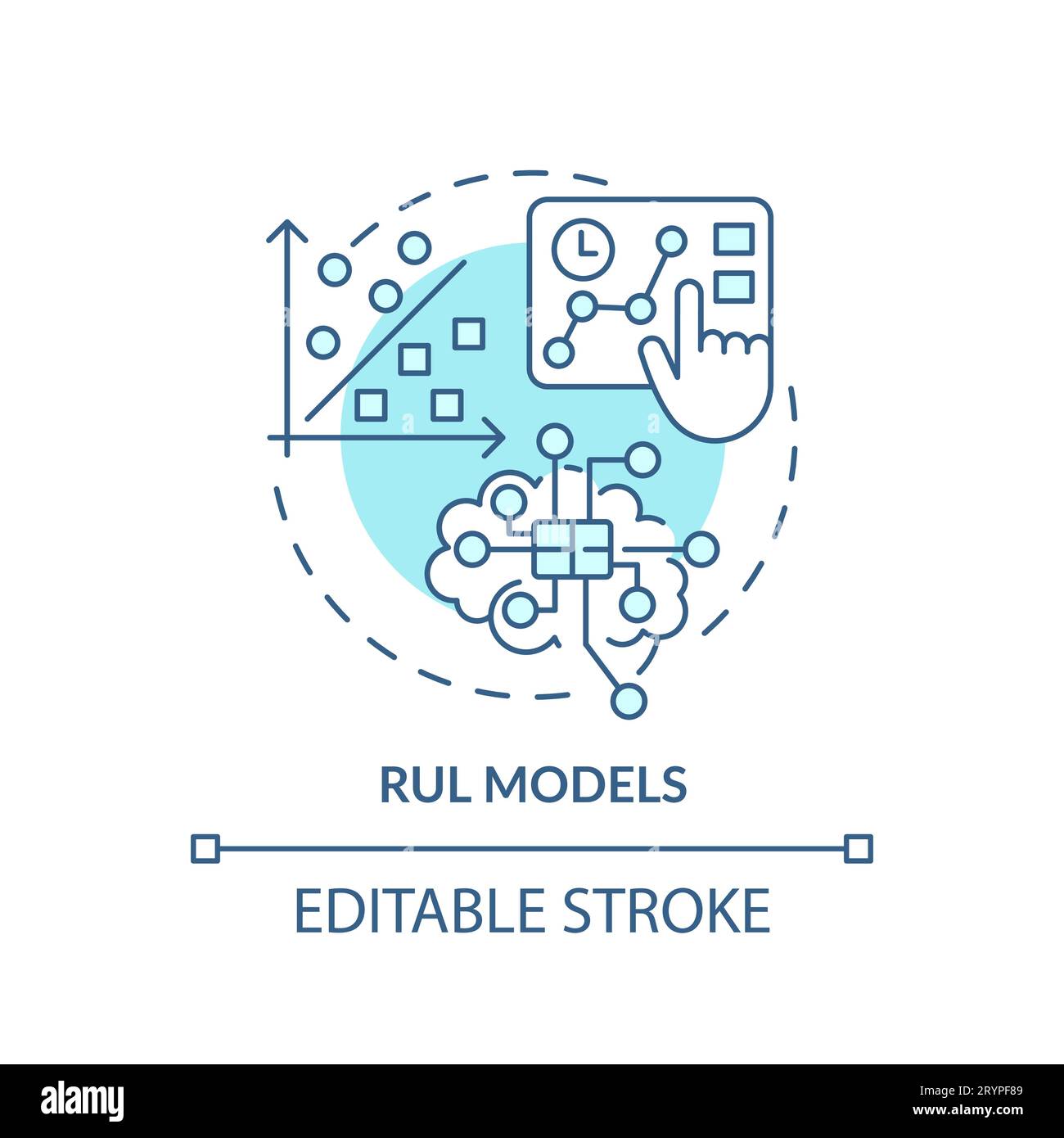